This guest post was written by MadTech industry veteran Therran Oliphant
What is Orchestration?
Whenever I start talking about orchestration, the thought bubble seen over most people’s heads is probably best described by the young Georgia poet, Gunna’s, hook to his popular song with a title I can’t write in this forum. Let’s just say it’s a three-word phrase for confusion that ends with, “you mean?” The reason for the confusion (especially in the marketing world), is because orchestration is a set of complex tasks involving the coordination of disparate technologies that is seamless when done correctly. It also doesn’t help that these processes are below the line for most non-technologists.
More generally, orchestration is the organization and supervision of multiple computer systems, applications, and/or services to string together normally disconnected tasks to execute a larger workflow or process. In marketing, we often describe end-to-end processes through the lens of automation. While automation is necessary, it’s the wing to the passenger plane that is orchestration, soaring through the sky.
For marketers, orchestration is as much a determinant of success as a strong campaign strategy or holistic measurement framework. This is because marketers’ tech stacks contain multiple components that need to work as a systematized unit of top-to-bottom tasks. Tasks that each play a pivotal role in sound and performant marketing campaigns.
Orchestration types
Given that this is more complex than a Rube Goldberg machine, let’s break it down into types. Each orchestration type has its own defining characteristics, and together, they offer a comprehensive insight into the array of orchestration options available. These can include but are not limited to journey orchestration, docker orchestration, and process orchestration.
As this post is aimed at marketers, we’ll focus our discussion on customer data orchestration and the technical components required at each layer. Note that technical folks generally associate orchestration layers with the coordination of multiple API services to create a single API request. In our case however we’ll define “layers” as the vertical layers of the tech stack. If it helps, think of a wedding cake.
Data orchestration for marketers
For marketers, orchestration means organizing and pipelining the volumes of data that come through the digital interactions and external partnership. The goal is to efficiently collect, enrich, and translate data across disparate platforms to better communicate with consumers and measure the efficacy of those communications. These relationships are often represented in schematics that depict the flow of data in processes set up by engineers. Below is an example of a diagram that martech teams can make to guide engineers, highlighting the specific layers of the stack that require integration (Figure 1).
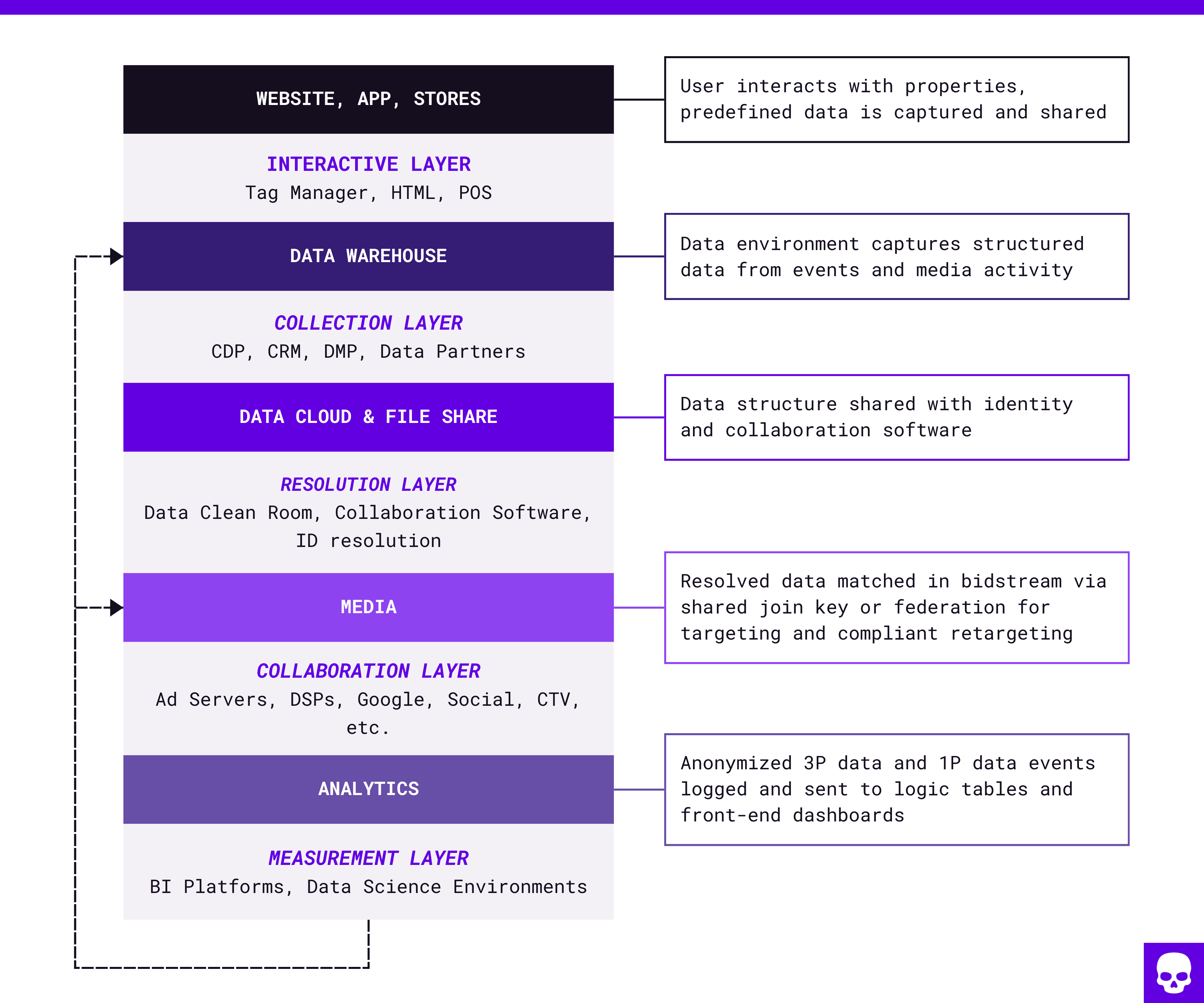
Figure 1 – Orchestration layers in an advertising technology stack
Orchestration layers in an advertising technology stack
Interactive layer
This layer comprises the onsite systems that govern the interactions between the brand and the client (e.g. site, app). These tools can include A/B or multivariate testing software, customer relationship management (CRM) tools, and content management software (CMS). They require rules and automation to tailor the site/app experience so that it’s consistent with brands’ desired communications environment.
Thus, the first layer of orchestration involves defining the data variables collected when a site visitor triggers an event. The corresponding software collects data correlating to the defined variables – commonly orchestrated end-to-end in data taxonomies and naming conventions pushed to all environments the brand interacts with. These events should be connected according to the orchestration parameters in the next layer of the stack.
Collection layer
This layer of the stack serves as an initial point of governance to make sense of the events that take place at the interactive layer. These components are integrated into websites, mobile apps, and even real-world environments. Examples include customer data platforms (CDP) and CRM systems, and data onboarders.
The main purpose of the collection layer is to connect visitation to the previously defined variables in order to either 1) provide insight on the consumer journey or 2) match these data points to exogenous knowledge sources. Today, the utility of the collection layer depends on marketers’ proficiency at utilizing first party collection tech (first party cookies, emails, customer data integrators) as join keys so that the data is usable at the next layer
Resolution layer
Now that the data has been defined and collected, it’s time to get some boxes and bubble wrap so that the data can move. Thus far, data has mostly remained in on-premise (on-prem) platforms that are either built or owned by the brand. But platforms like CDP’s and tag managers also publish data to external locations to deliver marketing solutions beyond the on-prem environment.
Marketers need to orchestrate this data with the right schema, cadence, and parameters to their partners. The resolution layer typically consists of API’s, cloud storage, enterprise data warehouses, identity partners, and the egress for some knowledge layer solutions. We could also call this stage data usability, as the data is now delivered to destinations that can deploy it to target ads, map to audience segments, or publish to dashboard logic tables to deliver visual insights. To operationalize this, privacy compliance, operational commands, and predefined mechanics/logic need to be orchestrated.
A quick intermission
So far, we’ve discussed customer interaction, data collection, and resolution to stage it for usage. Many marketers don’t take orchestration past this technical point. There was a time when this was enough, as once a third party cookie sync took place, audiences could be actioned on while ad tags collected all generated data at the log level and shared back to a campaign manager (often ad servers like Google Campaign Manager 360). The data in these logs were then used to inform other platforms such as data management platforms (DMPs) or multi-touch attribution (MTA) models. Today however, this is simply insufficient as most data is first party in nature and must be intentionally enabled by the brand at every step.
Now back to our regularly scheduled programming.
Collaboration layer
At this point, data has landed and has been organized into a usable format. It’s now necessary to get your data from your warehouse, CDP, analytics solution, or publisher partner account into the relevant data platform and/or inventory bid stream to match to users. This creates optionality, as the marketer should be comfortable that the data is informed with logic, privacy compliant, and can be enriched with additional signals for targeting, customer insights, or measurement.
The platforms at this stage are largely dependent on the use case(s) the marketer has in mind for the data. Technology types include but aren’t limited to data clean rooms, activation integrators, business intelligence dashboards, RTB platforms, and even social and publisher platforms. The data needs to inform these platforms to match your targeting parameters, campaign goals, and/or marketing objectives.
Measurement layer
Once the data leaves the collaboration layer, it can be used to interact with prospective customers, existing product users, as well as high value users via retargeting. It can also be analyzed using insights tools to inform which audiences to suppress to mitigate ad wastage. However, when humans see or interact with an ad (or are suppressed from seeing one), the data is enriched with additional fields. Data orchestration methodology takes this into account so that the schema fits our data capture model.
In other words, the taxonomy that was put in place at the interactive layer can now be used to process and integrate data into a knowledge framework. This should correlate with the naming conventions for the variables set up at the interactive layer so that marketing activity can be labeled and measured for impact. The insights that are produced can then be (re)deployed for data orchestration activities further upstream as the cycle repeats.
Worth the effort
To recap, we’ve established that orchestration encompasses a more complex and comprehensive set of processes than automation, which are point solutions for mechanizing specific tasks. These orchestration processes are composed of layers and must be properly configured to ensure efficient operation of the overall system. If not set up correctly, the system may break down, potentially compromising marketers’ campaigns and measurement frameworks. This can lead to errors, data paucity, and additional costs and time spent on quality assurance by data governance and analytics teams.
Additionally, we’ve explored how data traverses these layers, emphasizing the need for it to be stored and shared in a way that allows marketers to effectively interpret it for their desired use cases. Furthermore, the resulting use cases need to be measured in a manner that corresponds with the data collection and storage methods. One aspect we haven’t touched on is how different types of system integrations can either facilitate or obstruct the orchestration process, but that’s a discussion for another time.
So the next time your MarTech Manager or Data Engineer mentions resolution complexity, taxonomy, or schemas, you’ll understand that it’s all part of a larger orchestration framework. Although orchestration can be challenging, success is attainable with a well-defined and organized plan that aligns with marketers’ objectives. Understanding the specific objectives for your data at each orchestration layer is crucial. This clarity can provide a more seamless experience for customers, stronger marketing performance, and improved operational efficiency.