A version of this article was published in Campaign Asia on February 6, 2025.
Unless you’ve been living in an underground bunker without internet access, you’ve likely heard about DeepSeek in recent weeks. As one of those rare tech developments that has captured mainstream attention, the surge in interest in what’s objectively a complex topic has led to a wide range of narratives. For brands and advertisers, separating signal from noise and understanding the implications can be challenging.
Let’s start with the most important takeaway: DeepSeek is unlikely to have much (if any) direct impact on the marketing industry as it doesn’t unlock any AI-driven marketing use cases beyond what’s already possible with existing AI models. That said, its broader impact on accelerating AI development and adoption will lead to meaningful benefits across all sectors, including marketing.
In this article, I’ll provide some high-level background to help readers understand and contextualise the aspects of DeepSeek that are most material to its broader impact. We’ll do so by first walking through what DeepSeek is and why their AI models are significant. Next, we’ll explore the significance of DeepSeek in the wider AI scope and how this will impact consumers and businesses including those in our industry. Again, these benefits aren’t immediate or direct, but the implications should become apparent for readers as they progress through the article.
Lastly, DeepSeek obviously has implications beyond the technology itself with material impact on US/China geopolitics, chip regulations, Nvidia/tech stocks, and more. For the purposes of this article however, we’ll keep the focus on areas that will most impact marketers.
What is DeepSeek and why is it all over the news?
What is DeepSeek
DeepSeek is a Chinese AI company owned and funded by a Chinese Hedge fund named High-Flyer. With ~150 employees and growing rapidly, they are not a “side project” as described by some, but a serious AI lab that has been on analysts’ radar since releasing their V2 model in May 2024. The company made headlines near the end of January with their free chatbot app powered by two large-language models (LLMs): DeepSeek V3, their general-purpose foundational model, and DeepSeek R1, their specialised reasoning model built from V3.
Technical innovations
On a technical level, DeepSeek is significant as they achieved model performance comparable to those from leading Western IA labs (e.g. OpenAI, Anthropic) despite US chip sanctions limiting access to the most advanced Nvidia chips. In AI parlance, there are two terms to know when it comes to chip capabilities:
COMPUTE – The raw processing power required to train models and perform operations
MEMORY – The ability to hold and organise data while working on a task
Up to now, models have improved largely through increasing compute, memory, data and model size. Due to the chip sanctions however, DeepSeek had to work with chips that were memory constrained. This forced efficiency led to them developing breakthroughs in how AI models can be optimised, representing an alternate path from the “bigger is better” paradigm of simply throwing more resources at the problem.
Cost efficiences
These breakthroughs have led to significant cost efficiencies. Now this is a big deal, but the headlines of DeepSeek “doing for $6M what it took OpenAi $1B to do” is a misrepresentation and false. LLM costs can largely be split into two categories:
TRAINING – Costs of developing and training the model. This includes everything from infrastructure and compute/memory (i.e. GPUs), R&D, and actual model training runs.
INFERENCE – Costs of a model taking an input (i.e. prompt) and outputting a response. This can be consumers/businesses using the completed model or AI labs using existing models to output data to train new models.
The reported $6M ($5.576M to be exact) refers to only the final training run for the V3 model (which DeepSeek states in their technical paper) and excludes all other costs to reach that point. So no, V3 or R1 wasn’t developed from scratch for $6M.
On the other hand, DeepSeek’s cost efficiencies for inference (as a result of the aforementioned innovations) should be getting much more attention than it has. Based on API pricing, inference costs for DeepSeek models are orders of magnitude cheaper than that of comparable from Western competitors. Decreasing inference cost is a significantly more important enabler of AI permeation across applications and industries (including marketing/advertising).
Open weights
Another key differentiator is DeepSeek’s approach to open weights. While R1 may not be the absolute best model available, it’s considered the leading “open weights” model. Open weights? Yes, technically speaking, their models aren’t open source. Instead DeepSeek shares their models’ “weights”, but not everything that went into arriving at those weights (e.g. training data, process, etc.). They went a step further and released their models with MIT permissive licensing and detailed technical documentation, making it easier for businesses and developers to experiment with and integrate their technology.
What does this mean for brands and advertisers?
For brands and advertisers, the most significant impact will come from how DeepSeek catalyzes broader AI development and adoption. Taking a step back, the “AI stack” can be visualised as follows (Figure A).
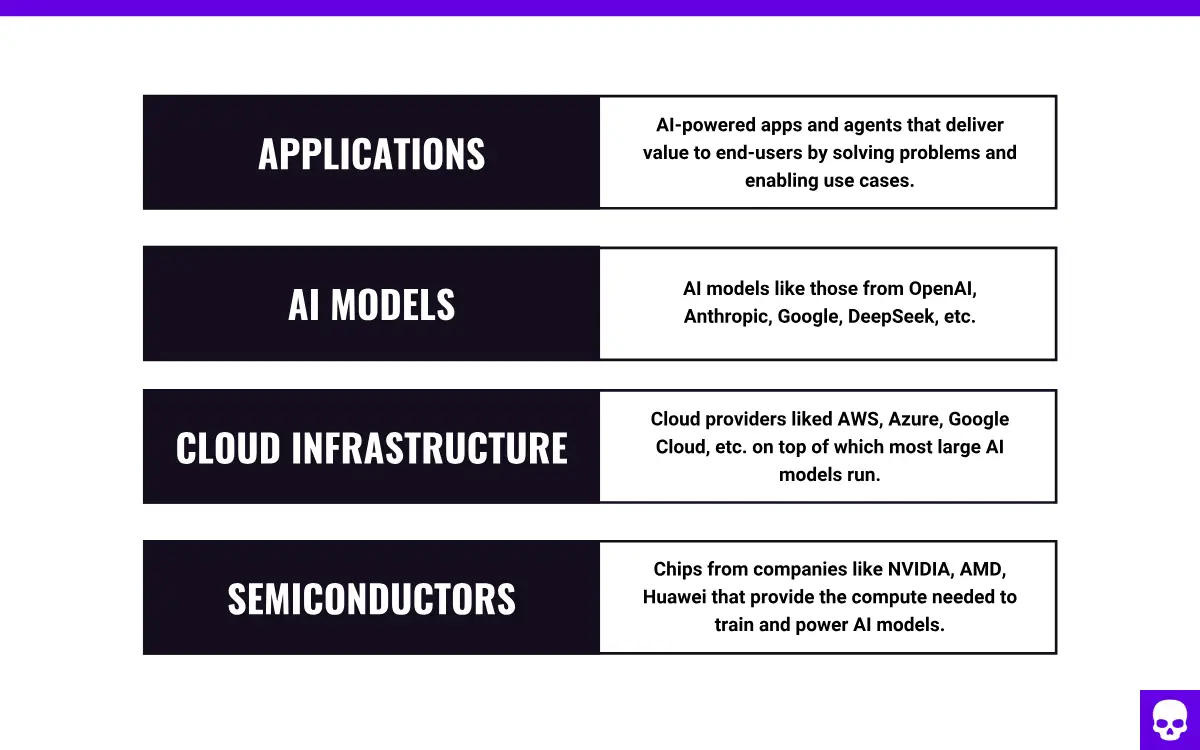
Figure A – High level AI stack
Over time, as the AI models layer becomes increasingly commoditised, differentiation will sit at the application layer. This is also where current and future AI-based marketing and advertising businesses will sit (Figure B).
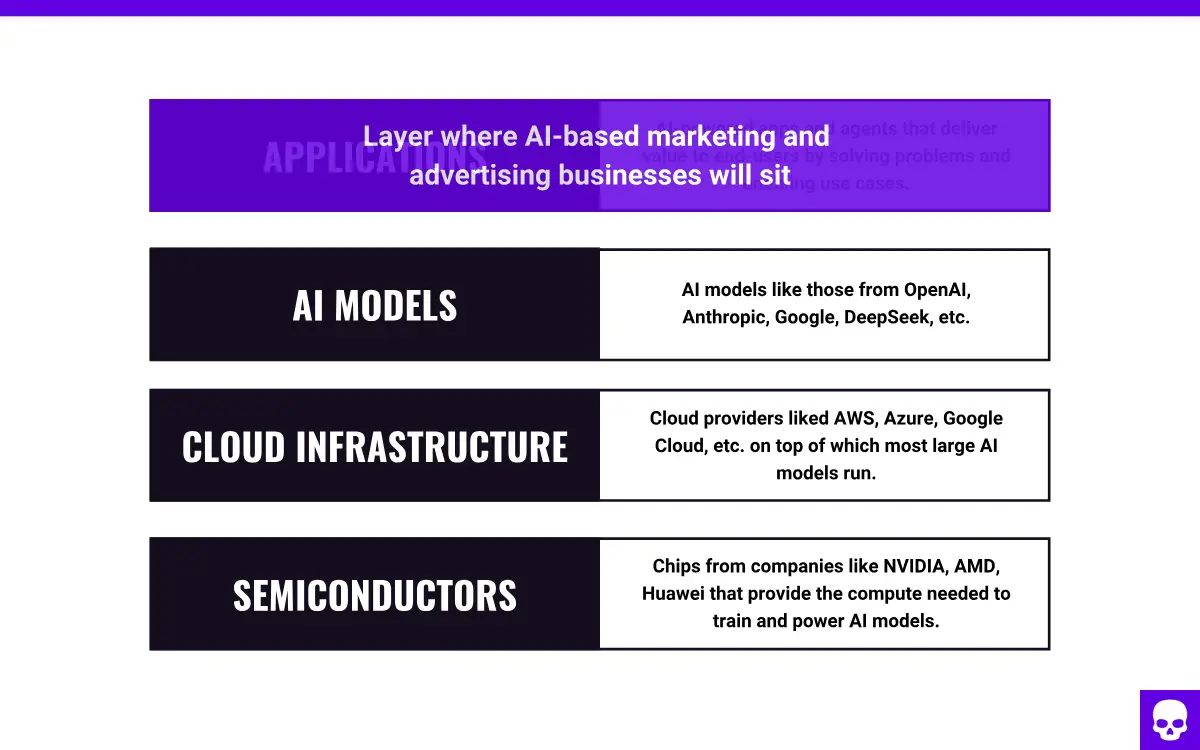
Figure B – AI-based marketing/advertising businesses within AI stack
As the marginal cost of inference continues to decrease, the economic viability and scalability of these businesses will skyrocket. This in turn, will dramatically increase AI-driven innovation and utility for the marketing industry. Using the past to inform the present, this is similar to how decreasing marginal costs for CPUs, internet and cloud computing enabled modern digital marketing.
The open weights + permissive licensing approach serves as a force multiplier for AI progress by lowering barriers to entry and enabling more customised solutions. This democratization benefits startups and SMBs while opening possibilities for domain-specific adaptations, including marketing applications. It also addresses privacy concerns, which is especially relevant as the DeepSeek app objectively collects more personal data than Western AI labs (see figure C for summary).
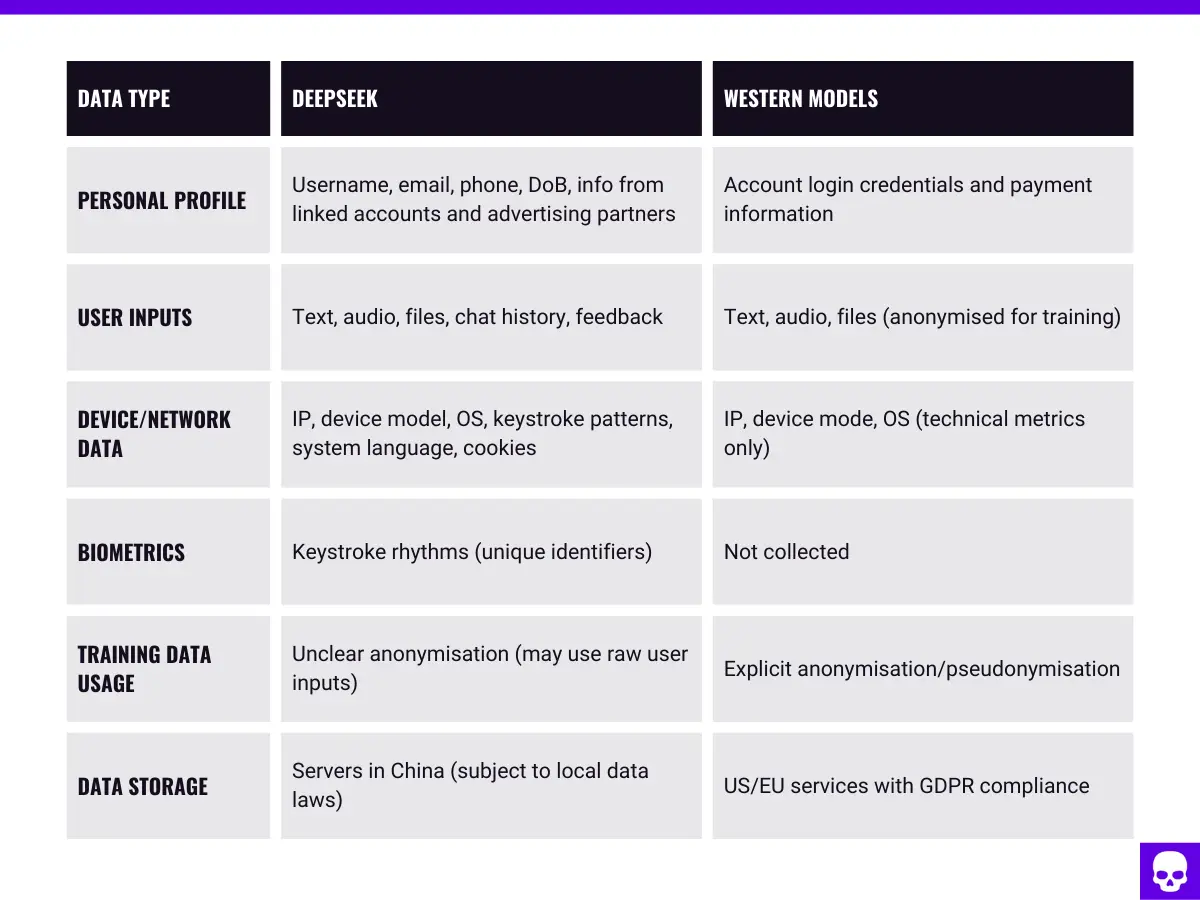
Figure C – Personal data handling comparison
As one might imagine, there are many who are uncomfortable with DeepSeek’s data privacy policies and the idea of sending their data to servers in China where it’s subject to local data laws. The good news is that while DeepSeek’s app is the most direct way to access their models, it’s not the only way. Users can run them locally or access them through apps/platforms (e.g. Perplexity, HuggingFace, Azure) with servers outside of China, preventing unwanted data sharing and PRC-related censorship.
DeepSeek’s emergence has intensified competition in AI, with China demonstrating its ability to compete with US leadership in the field. This has already prompted responses from OpenAI and Meta, who are accelerating development of their next models. More importantly for businesses, DeepSeek’s cost efficiencies have triggered pricing responses from Western labs, which will further catalyse development at the application layer where brands and advertisers stand to benefit most.
As the AI landscape continues to evolve, the indirect benefits of DeepSeek’s innovations (lower costs, increased competition, and broader access to AI capabilities) will likely have a more lasting impact on marketing and advertising than any direct applications of their specific models.